Science cannot get a decent break these days. Scientists around the world have even taken the unusual step of organizing a “March for Science” (on April 22) to defend their work, and the scientific view of the world, against some political ideologies of the far right (or alternative right). They had good reasons to do so, but the contempt of today’s far right for science is not per se a novelty: fascists from all times and places always disparaged science, because fascists believe in violence, not in arguments, and they use force, not facts, to prevail. In that, today’s fascists are not different from their twentieth century predecessors. But let’s forget about them—the fascists—for a moment, and for the sake of the argument, let’s turn out attention to the scientific community instead. Which science is supposed to be under threat, precisely? Modern, inductive, experimental, inferential science—the science of Galileo and Newton: the science we all studied at school—may appear to be the prime target of today’s alt-right. But that is far from being the only science available on the marketplace of ideas. Since the first formulations of Heisenberg’s quantum mechanics in the late 1920s, and more powerfully since the rise of post-modern philosophy in the late 1970s, several alternatives to modern science have been envisaged and discussed by philosophers and scientists alike, and today the theories of non-linearity, complexity, chaos, emergence, self-organization, etc. do not seem to be under any threat at all. In fact, most of these theories never had it so good. That’s because some post-modern ideas of complexity and indeterminacy have been revived, and powerfully vindicated by today’s new science of computation.
Thirty years ago anyone could have argued that Deleuze and Guattari’s theory of science was fake science (and some did say just that). Today, on the contrary, few can deny that advanced computation follows a post-scientific method that is way closer to Deleuze and Guattari’s worldview than to Newton’s. 1 And no one can deny that, when used that way, and specifically when putting to task a range of processes loosely derived from, or akin to, some post-modern ideas of complexity, computers today work splendidly well, and produce valuable, usable, effective results. Let’s face it: what many still like to call Artificial Intelligence, machine learning, or whatever, is nothing artificial at all. It is just a new kind of science—a new scientific method. In fact, if we think of science as modern science exclusively, then computation is a new, revolutionary, post-modern and post-scientific method: it is, in fact, the most drastic alternative to modern science ever, because, unlike many obscure ideological proclamations by any anti-modern wacko, of which the twentieth century produced plenty, computation (or AI) today can be proven to work.
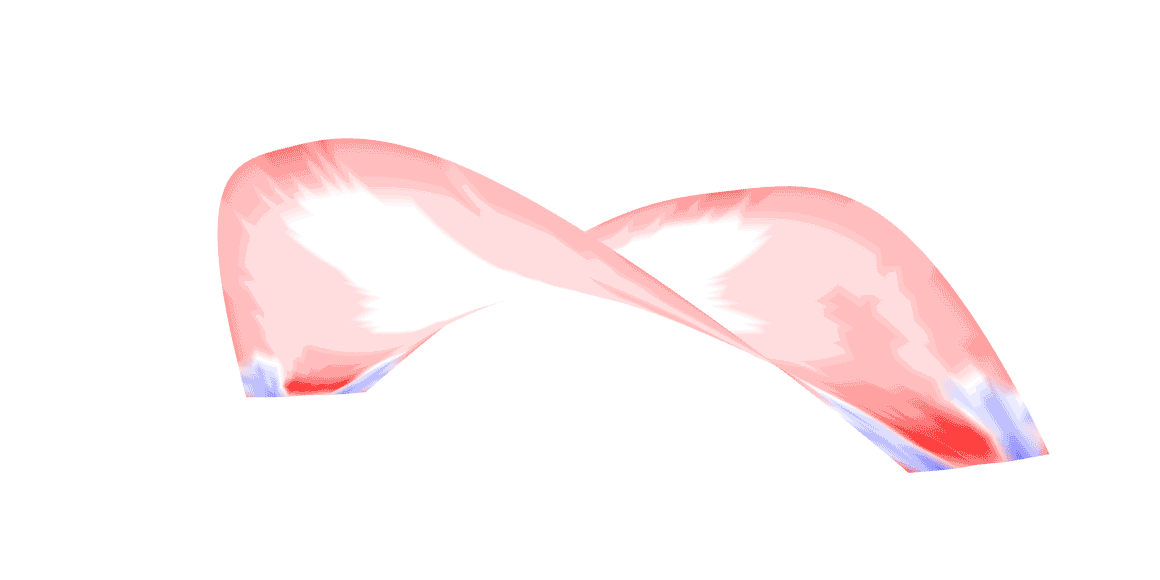
This render uses the software Octopus to find the optimal height and width of a concrete shell bridge based on the deflection, weight, and height.
And this is where the design professions come into play. The design professions were among the earliest and most enthusiastic adopters of digital technologies one generation ago, and they have recently moved on to testing new computational tools for simulation and optimization. These are no longer just tools for making: they are primarily tools for thinking; and the first consequences of the adoption of these inchoate AI instruments in design, in design theory, and in design education, are already perceivable. These early signs often show surprising affinities with the anti-scientific ideology of today’s alt-right. Yet most designers engaged in computational experiments, to the best of my knowledge, are not fascist at all; moreover, the alt-right has not shown to date any interest in generative algorithms, nor can it be plausibly argued that the alt-right is a degenerate political avatar of French post-structuralist philosophy. This convergence of ideals between otherwise ostensibly unrelated doctrines and disciplines is therefore most puzzling, and it invites scrutiny. This is where I suggest we need to stop for a minute, take a deep breath and have a better look at what is going on.
Modern science used to be based on the observation of factual evidence: on that basis, through a process of comparison, selection, generalization, formalization and abstraction, experimental science arrives at general statements (often in the format of mathematical formulas) we use to predict future phenomena. At the same time, scientific formulas or laws also offer causal links between some variables in the phenomena they describe: these cause-to-effect relationships offer clues to interpret these phenomena, and to make some sense out of them. So the simple formula used to calculate the speed of impact on the ground of an object in free fall, derived from Newton’s law of gravitation, relates that speed to the acceleration of gravity and the height of the fall, thus making it clear, for example, that the way a solid falls to the ground is not related to the date of birth and horoscope of the scientist performing the experiment—nor, indeed, to anything else not shown in that formula. Built on similar premises, statics and mechanics of materials are among the most successful of modern sciences. Structural designers use the laws and formulas of elasticity to calculate and predict the mechanical resistance of very complex structures, like the wing of an airplane, or the Eiffel Tower, before they are built, and in most standard cases they do that using numbers, not by making and breaking physical models in a workshop every time anew. That’s because those numbers—those laws—condensate and distil in a few lines of neat mathematical notations the lore acquired through countless experiments performed over time.
This is where the unprecedented power of today’s computation has already proven to be a game changer. Given today’s Big Data environment, we can reasonably expect that at some point in the near future almost unlimited data collection, storage, and retrieval will be available at almost no cost, and that almost infinite records of all sorts of events may be indefinitely and indiscriminately kept and accrued, without the need for any systematic (or “scientific”) selection, sorting, or classification whatsoever. What is today already a reality in the case of user-generated data (which is simply kept after use, and never discarded) may extend to the monitoring and tracking of all kinds of physical and material phenomena, such as the functioning of an engine, the bending of a skyscraper under wind loads, or global and local weather patterns. In this ideal condition of superhuman access to data, predictive science as we know it will no longer have any reason to exist, as the best way to predict any future event (for example, the development of a weather pattern) will be to search for a recorded precedent of it; such precedents will simply be expected to repeat themselves in the same conditions. Omniscience per se may not be within reach anytime soon, but our current data opulence already allows for very similar information retrieval strategies, which have started to replace analytic, deductive calculations for many practical purposes and in many applied sciences. As I discuss at length elsewhere, this is happening—although, for some reason, no one is saying so too loudly. 2
Likewise, and in the same spirit, so to speak, if the event we are trying to predict is unprecedented, we can use the immense power of today’s computational tools to simulate as many fictional precedents as needed, thus virtually recreating and enabling the same heuristic strategy just described: among so many precedents, never mind if real or virtual, we shall at some point find one that matches the problem at hand. Thus, for example, in the case of structural design, we can calculate the resistance, rigidity, or weight of any given structure designed almost at random, and then, using computational simulations, we can tweak that structure, and recalculate it as many times as needed until we find one that has the required resistance, rigidity, or weight.
This, it must be noted, is not very different from what engineers always did—except that calculating a structure by hand takes time, so the number of variations that a designer could recalculate thirty years ago, for example, was limited. Given these practical limits, designers of old did their best to come up with a good idea from the start. And engineers could do that because the laws of rational mechanics are, in a sense, transparent: by the way they are written, they provide a causal interpretation of the phenomena they describe, and they allow designers to infer some understanding of the forces, stresses, and deformations at play in the structures they design. But today, computers can recalculate endless variations so fast that the structure we start with is almost irrelevant: sooner or later, computational simulations will come up with what we need anyways. Traditional, pre-industrial craftsmen, who were not engineers, and did not use math, had no alternative to iterative trial and error: they made a chair, for example, and if it broke, they made another, and then another, until they made one that did not break; if they were smart, they could also learn something in the process. Today, we can make and break on a computer screen more models in a minute than a traditional craftsman made and broke in a lifetime. The difference is, we are not expected to learn anything in this process, because the computer does it in our stead. We call this optimization.
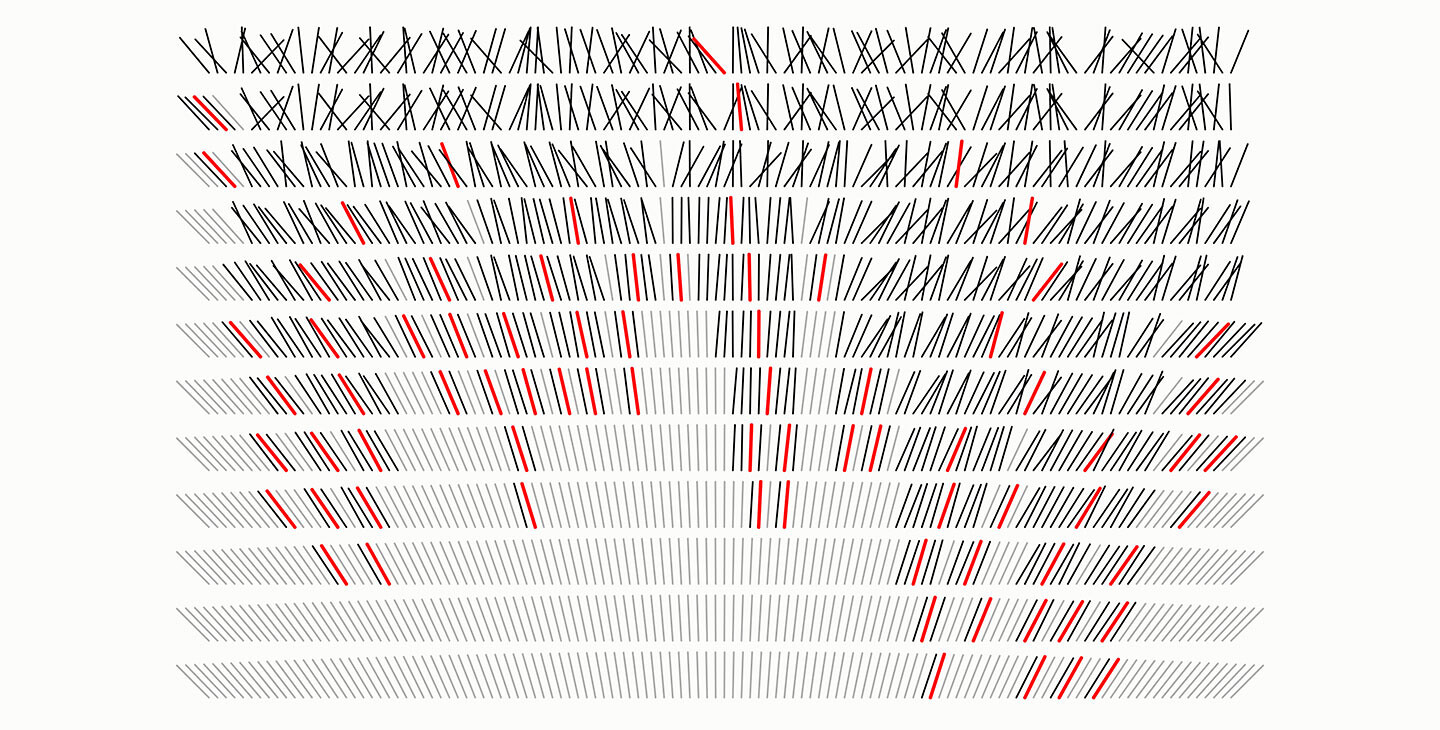
A graphic rendition of the sorting algorithm ‟Quicksort” by Mike Bostock, former editor of data visualization for the New York Times.
Indeed, as picking and choosing among a huge number of randomly generated variations still takes time, it makes sense to let computers make that choice, too; which some think is the same as asking the machine to exert some form of intelligence. But there is nothing intelligent in what computers do to carry out this task, and in the way they seem to learn from doing. Computers win by brute force—not by guile but by strength. Computers try at random endless variations of the parameters they are given, blindly shooting in all direction, so to speak; when they see they hit something, somewhere (i.e., that some parametric values lead to better results for the specific output that is being tested), they refocus on those values, excluding all others and then restart, ad libitum atque ad infinitum—or until someone pulls the plug and stops the machine. No convergence of results is expected to emerge from this open-ended, almost Darwinian evolutionary process, and most of the time none does. At some point, the designer will see that the machine starts to churn out results that appear to be good enough for the purpose at hand, and that’s it: end game. No need to know why this one particular structure on the screen works better than two billion others just tried and discarded: nobody knows that. Computers do not play the causality game, because they are so powerful they don’t need it. This is why we can use them to solve problems that are too complex for our mind, and even our imagination, to grasp.
Whether we like it or not, AI already outperforms us and outsmarts us in plenty of cases, and AI can already solve many problems that could not be solved in any other way. But computational machines do not work the way our mind does, and they solve problems following a logic that is different from our logic—the logic of our mind, and of almost all experimental sciences we derived from it. Computers are so fast that they can try almost all options on earth and still find a good one before they run out of time. We can’t work that way because that would take us too long. That’s why, over time, we came up with some shortcuts (which, by the way, is what method originally meant in Greek). This is what theories are for: theories condense acquired knowledge in user-friendly, short and simplified statements we can resort to—at some risk—so we do not have to restart from zero every time.
Yet theories today are universally reviled—just like modern science and the modern scientific method in general. Think of the typical environment of many of today’s computational design studios: the idiotic stupor and ecstatic speechlessness of many students confronted with the unmanageable epiphanies of agent-based systems, for example, may be priceless formative experiences when seen as steps in a path of individual discovery, but become questionable when dumbness itself is artfully cultivated as a pedagogical tool. Yet plenty of training in digitally empowered architectural studios today extols the magical virtue of computational trial and error. Making is a matter of feeling, not thinking: just do it. Does it break? Try again… and again… and again. Or even better, let the computer try them all (optimize). But the technological hocus-pocus that too often pervades many of today’s computational experiments reflects the incantatory appeal of the whole process: whether something works, or not, no one can or cares to tell why.
Unfortunately, this frolic science of nonchalant serendipity is not limited to design studios—where, after all, it could not do much harm in the worst of cases. This is where our dumbness, whether ingenuous or malicious, appears to be part to a more general spirit of the time. For the same is happening on a much bigger scale in the world at large, out there: as we have been hearing all too often from the truculent prophets of various populist revolutions in recent times, why waste time on theories (or on facts, observation, verification, demonstration, proof, experts, expertise, experience, competence, science, scholarship, mediation, argument, political representation, and so on—in no particular order)? Why argue? Using today’s technology, every complex query can be crowdsourced: just ask the crowds. Or even better, just try that out, and see if it works.
This where the alt-right rejection of factual argument, the ideology of post-modern science, and the new science of computation appear to be preaching the same gospel, all advocating, abetting, or falling prey to the same irrational fascination for a leap in the dark. For the fascists, it is the leap of creative destruction, war, and dictatorship; for po-mo philosophers, it is the leap and somersaulting of a non-linear, “jumping” universe; for the alternative science of computation, it is the leap to the wondrous findings of AI, or to the unpredictable “emergence” of supposedly animated, self-organizing material configurations (never mind that the growth of cellular automata, in spite of its mind-blowing complexity, is perfectly deterministic, and never mind that most purpose-built structures made of inorganic materials can be at best as animated as a cuckoo-clock). But if fascism and post-modern vitalism are ideologies, AI is a technology. True, computers work that way, but we don’t; and having humans imitate computers does not seem any smarter than having computers imitate us. Computers can solve problems by repeating the same operation an almost infinite number of times. But as we cannot compete with computers on speed, trial and error is a very ineffective, wasteful, and often dangerous strategy in daily life. Computers don’t need theories to crunch numbers, but we need theories to use computers. Let’s keep post-human science for AI, and all other sciences for us.
Take for example the distinction between the “smooth” science of pre-industrial craft and the “striated” science of modern mathematics in chapter 12 of A Thousand Plateaus, first published in French in 1980. Deleuze himself would eventually contradict some of these arguments in his book on Leibniz, The Fold, and the Baroque (1988), where differential calculus is seen as the successful merger of the two sciences (as infinitesimal numbers are used to emulate, reenact, and manipulate the smooth continuity of natural materials and geometric operations). Both arguments were extremely influential in the rise of early digital design theories in the 1990s.
See in particular my book The Second Digital Turn: Design Beyond Intelligence, forthcoming with the MIT Press in September 2017.
Artificial Labor is a collaboration between e-flux Architecture and MAK Wien within the context of the VIENNA BIENNALE 2017 and its theme, “Robots. Work. Our Future.”
Category
Subject
Artificial Labor is collaborative project between e-flux Architecture and MAK within the context of the VIENNA BIENNALE 2017.